Open miladfa7 opened 4 years ago
Hi @miladfa7,
I experienced this problem when training with 1 class too. However, I had my labelled images in separate tfrecords rather than 1 large tfrecord.
This issue arises because certain data(labelled images) are "corrupted"(which causes the loss to go to NAN). In my case, since I had labeled images in separate tfrecords, certain tfrecords were "corrupted". I noticed this because some tfrecords caused nan values while others didn't. So what I would suggest is having all of your labeled images in individual tfrecords. Then create a script that would iterate through each tfrecord(in other words , run the training on 1 tfrecord at a time) to find the corrupted tfrecords. In my case, there weren't a lot of corrupted tfrecords(around 30 for 2000 images). So I would suggest first iterating over batches of tfrecords(e.g. 25 or 50 at a time) and then locate all of the batches that give an NAN loss value. After you have located all of the file batches that give NAN, iterate through all of them 1 by 1 to find the corrupted files. This worked for me, I got rid of the corrupted tfrecords, and the training went fine. I haven't figured out why certain tfrecords are "corrupted" but i just got rid of the ones that give NAN loss.
I would suggest looking at #103 and #86 for more info.
Also if you are training with yolo tiny can you update me on the mAP and accuracy of your algorithm. I only get around a 40 percent accuracy with 5000 images. I get a 65 percent mAP with 0.3 iou.
can you run this script and check if your dataset is in the proper format?
python tools/visualize_dataset.py --dataset ./train.tfrecor --classes=./data/voc2012.names
can you run this script and check if your dataset is in the proper format?
python tools/visualize_dataset.py --dataset ./train.tfrecor --classes=./data/voc2012.names
I executed this command
output : Drone, 1, [0.641026 0.617857 0.517949 0.414286]
The problem is that the bounding box is not drawn correctly...
I normalized the bounding box but didn't normalize the images. Could this be the problem?
When I don't normalize the bounding box, there are no bounding boxes in the output image
output: Drone, 1, [131.5 129. 251. 224. ]
@zzh8829 @Robin2091
I solved this problem https://github.com/zzh8829/yolov3-tf2/issues/135#issuecomment-569708156
output:
I training model with 1 class and Instead of sparse_categorical_crossentropy use binary_crossentropy(...) and change the num_classes FLAG on the train.py to 1 class.
python3 train.py --dataset ./train.tfrecord --classes ./data/voc2012.names --num_classes 1 --mode fit --transfer darknet --batch_size 10 --epochs 10 --weights ./checkpoints/yolov3-tiny.tf --weights_num_classes 80 --tiny
The loss output was negative values when I executed this command. (my dataset include 350 drones image)
What could be the reason?
another question is how to improve the accuracy of the detection new image?
first of all use train_dataset = train_dataset.repeat()
and same for validation.
nan
values happen if your annotations are not x_min, x_max, y_min, y_max
.
If your becomes negative, there's something really wrong.
Anyways, use a lower learning rate parameter, try 1e-4, because on 1e-3 u might overshoot.
@zzh8829 @Robin 2091 我解决了这个问题#135(评论) 产出:
1类训练模型,用二进制交叉熵代替稀疏分类交叉熵(.)并将列车.py上的num_class标志更改为1类。
python3 train.py --dataset ./train.tfrecord --classes ./data/voc2012.names --num_classes 1 --mode fit --transfer darknet --batch_size 10 --epochs 10 --weights ./checkpoints/yolov3-tiny.tf --weights_num_classes 80 --tiny
损失产出是负值时,执行此命令。(我的数据集包括350架无人机图像)
原因是什么?
另一个问题是如何提高新图像检测的准确性?
我执行以下命令
python3 train.py --dataset ./train.tfrecord --classes ./data/voc2012.names --num_classes 1 --mode fit --transfer darknet --batch_size 8 --epochs 10 --weights ./checkpoints/yolov3-tiny.tf --weights_num_classes 80 --tiny
但是我得到的是训练时的错误信息(“NaN值”)。
我的训练数据集中只有一个类别。(无人机探测)
此错误是否与将txt转换为tfRecord有关? 我在此基础上转换了我的数据集#41" 请帮帮我,谢谢。
I also encountered this problem. when the category is 1, the loss also appears Nan. later, I changed the loss function according to your method. later, the loss also appears negative. how can I solve this problem
I execute this command
python3 train.py --dataset ./train.tfrecord --classes ./data/voc2012.names --num_classes 1 --mode fit --transfer darknet --batch_size 8 --epochs 10 --weights ./checkpoints/yolov3-tiny.tf --weights_num_classes 80 --tiny
but I get is this error message while training("nan values" ).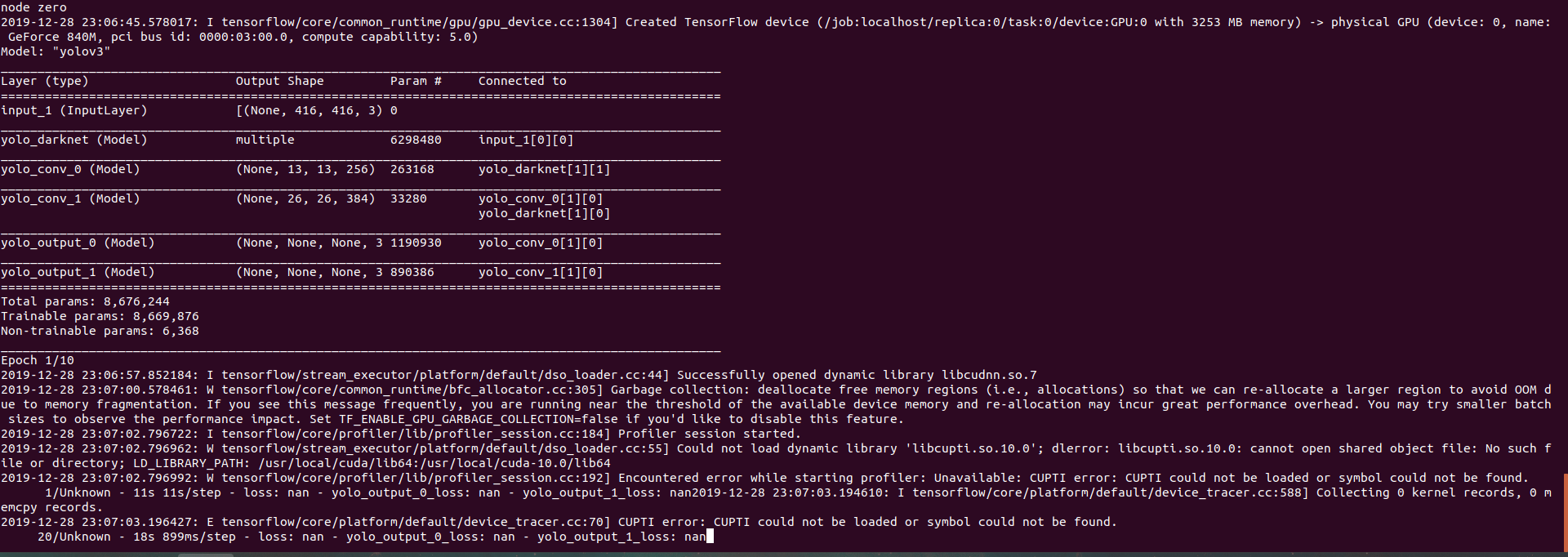
I only have 1 class in my training dataset.(Drone detection)
Do this error is related to convert txt to tfrecord? I converted my dataset based on this "https://github.com/zzh8829/yolov3-tf2/issues/41" please help me, thanks.