Dohyun Kim & Jihun Jeung
LOAD data를 WindowsGenerator를 이용하여, Model을 만들고 학습시키려함.
향후 Load data에 기상청 데이터 ex) weather data를 붙여 CNN_LSTM 모델을 적용해보려 함. (normalization을 적용함, )
Electricity data를 일별로 총합을 구해 total_sum.csv를 만듬
특정 구간에서 급격히 떨어지는 부분을 빼는 등 데이터 전처리 과정이 필요함
PV의 경우. 제한조건 및 objective function 등 필요한 수식을 만듬.
PULP library를 공부 중. 이를 적용 예정.
Spark를 이용해 PV Data과 load data를 input으로 전기요금을 계산해볼 예정 (?)
example data
timestamp | electricity | korea_electricity | temperature | ... |
---|---|---|---|---|
timestamp = [0] * length #initialization
for i in range(length):
timestamp[i] = time.mktime(datetime.datetime.strptime(weather_df['timestamp'][i], '%Y-%m-%d %H:%M').timetuple())
weather_df['timestamp'] = timestamp
#figure size
plt.rcParams['figure.figsize'] = [20, 5]
b, a = signal.butter(1, 0.3)
y = signal.filtfilt(b, a, xn)
plt.figure plt.subplot(1,1,1) plt.plot(t, xn, 'b') plt.plot(t, y, 'r') #lfilter plt.legend(('noisy signal','filtfilt'), loc='best') plt.grid(True) plt.show()
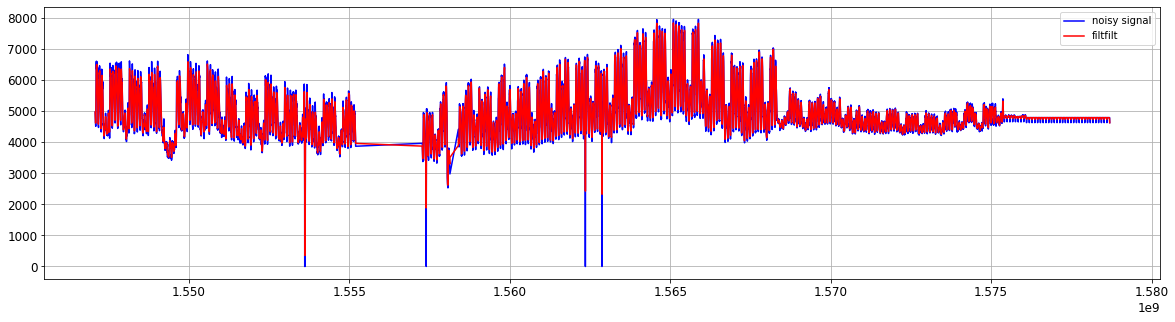
Figure. low pass filter
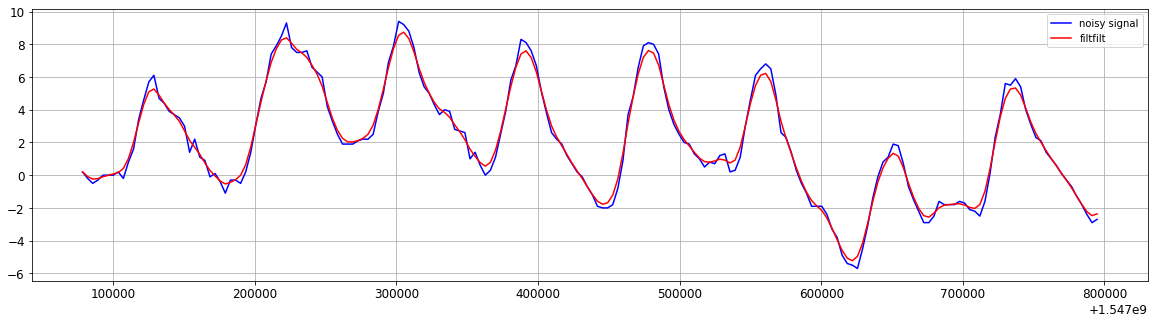
Figure. low pass filter (zoom-in)
- trim outlier (electricity < 2000)
df_data2 = df_data df_data2 = df_data2.drop(list(range(3739,3742)), axis=0) df_data2 = df_data2.drop(list(range(3595,3598)), axis=0) df_data2 = df_data2.drop(list(range(2472,2499)), axis=0) df_data2 = df_data2.drop(list(range(2288,2291)), axis=0) df_data2 = df_data2.drop(list(range(1813,1820)), axis=0)
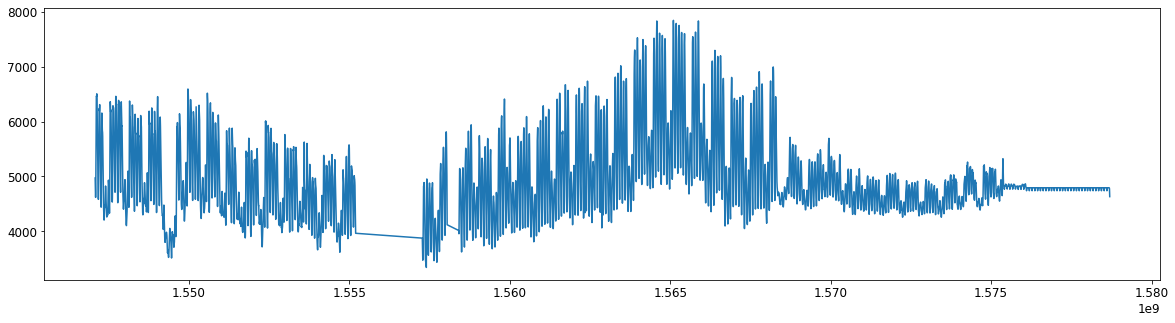
- correlation
corr = df_data.corr(method='pearson') print(corr) timestamp electricity ... 지면온도(°C) korea_electricity timestamp 1.000000 -0.011660 ... 0.127391 -0.055148 electricity -0.011660 1.000000 ... 0.454906 0.633428 year 0.278708 -0.041752 ... -0.173866 0.098974 month 0.840054 0.007057 ... 0.229869 -0.117772 date -0.001209 0.054572 ... -0.002976 0.047094 day -0.008416 -0.006461 ... -0.007596 -0.368987 time 0.002965 0.295113 ... 0.164734 0.321971 temperature 0.192824 0.380005 ... 0.921429 -0.064204 강수량(mm) 0.021677 0.045517 ... 0.031095 0.002487 풍속(m/s) -0.070830 0.230423 ... 0.196186 0.192416 풍향(16방위) -0.164018 0.165331 ... 0.238157 0.113418 습도(%) 0.176682 -0.071612 ... 0.007366 -0.176961 증기압(hPa) 0.197663 0.378798 ... 0.718443 -0.016517 이슬점온도(°C) 0.241461 0.280366 ... 0.728326 -0.120461 현지기압(hPa) 0.053933 -0.242467 ... -0.687093 0.092686 해면기압(hPa) 0.044979 -0.248686 ... -0.701145 0.093071 전운량(10분위) 0.032239 0.101038 ... 0.118865 0.055340 시정(10m) 0.148792 0.132798 ... 0.163565 0.055807 지면온도(°C) 0.127391 0.454906 ... 1.000000 0.019157 korea_electricity -0.055148 0.633428 ... 0.019157 1.000000
- feature significance
from sklearn.ensemble import RandomForestRegressor from matplotlib import pyplot
split into input and output
X = df_data.iloc[:, 1:] #timestamp y = df_data.iloc[:, 0]
fit random forest model
model = RandomForestRegressor(n_estimators=500, random_state=1) model.fit(X, y)
show importance scores
names = df_data.columns.values[1:] for name, score in zip(names, model.featureimportances): print(name, score) print('------------------------------------------------------')
electricity 4.8691318645328e-07
year 0.1641875308458571
month 0.8298849282469517
date 0.005836185661765732
day 4.891873361976034e-05
time 2.1977916794647093e-06
temperature 6.764042455895493e-07
강수량(mm) 2.687023373225491e-08
풍속(m/s) 2.711072603142201e-07
풍향(16방위) 1.3002849610985465e-07
습도(%) 5.492011958910649e-07
증기압(hPa) 3.6728833845211496e-06
이슬점온도(°C) 3.936398224801228e-06
현지기압(hPa) 1.4493666814833007e-05
해면기압(hPa) 1.099987486407661e-05
전운량(10분위) 8.007781093506881e-07
시정(10m) 9.965253005783227e-07
지면온도(°C) 3.428553540672571e-07
korea_electricity 2.855213455813887e-06
loss function= MSE
, optimizer=Adam
, learning rate=0.001
으로 설정 Input_window = 7
)로 다음날 (sliding_width = 1
) 24 hour를 예측(label_width = 1
)하는 모델을 만듬.keras tuner
는 TensorFlow 프로그램에 대한 최적의 하이퍼파라미터 세트를 선택하는 데 도움을 주는 라이브러리이다. BayesianOptimizer
은 gaussian process으로 적합한 hyperparameter tuning한다. max_trials=80
으로, objective='val_loss'
으로 설정하여 loss value가 가장 낮은 hyperparameter를 선정하였다.2018년 11월 1일 데이터 링크
#import libarary
from pulp import *
import tensorflow as tf
import pandas as pd
#load model
reconstructed_model = keras.models.load_model("/EnerGist_2021.07/LSTNet/elec_batch_129_453.h5", custom_objects = {'rse':rse,'PostSkipTrans': PostSkipTrans, 'PreSkipTrans':PreSkipTrans,'PreARTrans':PreARTrans, 'PostARTrans':PostARTrans} )
#predict
prediction_result = model_predict(reconstructed_model, 2018_11_01_data)
2018년 11월 1일 데이터 링크
#import libarary
from pulp import *
import tensorflow as tf
import pandas as pd
#load model
reconstructed_model = keras.models.load_model("/model_address", custom_objects = {'rse':rse,'PostSkipTrans': PostSkipTrans, 'PreSkipTrans':PreSkipTrans,'PreARTrans':PreARTrans, 'PostARTrans':PostARTrans} )
#predict
prediction_result = model_predict(reconstructed_model, 2018_11_01_data)
Library pulp
로 cost를 최소화해주는 BESS schedule을 찾아주는 linear optimization을 구현하였음. optimization(season, electricity_data, PV_data)
으로 사용가능하다. argument는 24시간 동안 사용한 electricity 값(e.g. electricity_t)과 PV 값(e.g. PV_t), 그리고 계절(e.g. 'summer')이다. 함수의 출력값은 total_cost
와 시간별 bess_value
이다.
>>> PV_t=[0,0,0,0,0,0,0,0.6,11.8,58.5,125.2,196.1,235.7,308.1,68.8,48,25,1.5,0,0,0,0,0,0]
>>> electricity_t=[4972.86446897, 4827.32813707, 4714.39951693, 4640.4637654 ,
4619.08798299, 4696.02395711, 4934.15191917, 5358.15019654,
5870.89139808, 6269.93405485, 6444.9726441 , 6461.49278899,
6454.26552802, 6488.01214138, 6506.49025023, 6471.5219868 ,
6409.99699496, 6273.83475456, 6020.27016168, 5780.28216133,
5637.47629486, 5505.49176825, 5340.39785949, 5166.06439475 ] #define electricity
>>> total_cost, bess_value = optimization('summer', electricity_t, PV_t)
>>> print(total_cost)
11904816.96244172
>>> print(bess_value)
[0.0, 0.0, 0.0, 0.0, 0.0, 0.0, 0.0, 0.0, 0.0, 0.0, 193.2964, 250.0, 0.0, 250.0, 59.50512, 180.68505, 0.0, 0.0, 0.0, 0.0, 0.0, 0.0, 0.0, 0.0]
''sh python optimization.py