Closed Jay-Patel-257 closed 1 year ago
Hi @Jay-Patel-257, thank you for submitting this demo! We will review it and let you know when it's up on our website.
Hi @Jay-Patel-257, great demo! We should be putting it up on the website early next week. Congratulations!
Hi @Jay-Patel-257, I made a few updates to the description of the demo. Please let me know if you're ok with the new description. Also, if you want to rephrase it and make it shorter it would be great.
The variational quantum eigensolver (VQE) is generally used for finding the ground state energy of a given hamiltonian. To find the kth excited state energy of the hamiltonian we need to run the VQE optimization process at least k+1 times. Each time we also need to calculate the hamiltonian again, taking into account the state of the previous iteration. Even after that, the accuracy decreases as the value of k increases. The Subspace Search VQE (SSVQE) algorithm is used to find the kth excited-state energy of a hamiltonian in two subsequent optimization processes. Research on a more generalized version of SSVQE, namely Weighted SSVQE, shows that by using the weights as hyperparameters we can find the kth excited-state energy in just a single optimization process. There are two variants of this algorithm: Weighted SSVQE to find kth excited state energy, and weighted SSVQE to find all energies up to the kth excited state.
Thank you!! @CatalinaAlbornoz I like the changes in the description, please go ahead with it!
Hi @Jay-Patel-257, I wanted to let you know that the demo is up on the PennyLane website! We will be promoting it tomorrow on the PennyLane Twitter. Congratulations!
Thanks a lot @CatalinaAlbornoz !!
General information
Name Jay Patel Siddharth Patel Amit Hirpara
Affiliation (optional) Pandit Deendayal Energy University
Twitter (optional) Jay Patel: The_Jay_Patel Siddharth Patel: Siddharth1India Amit Hirpara: am_hirpara
Image (optional)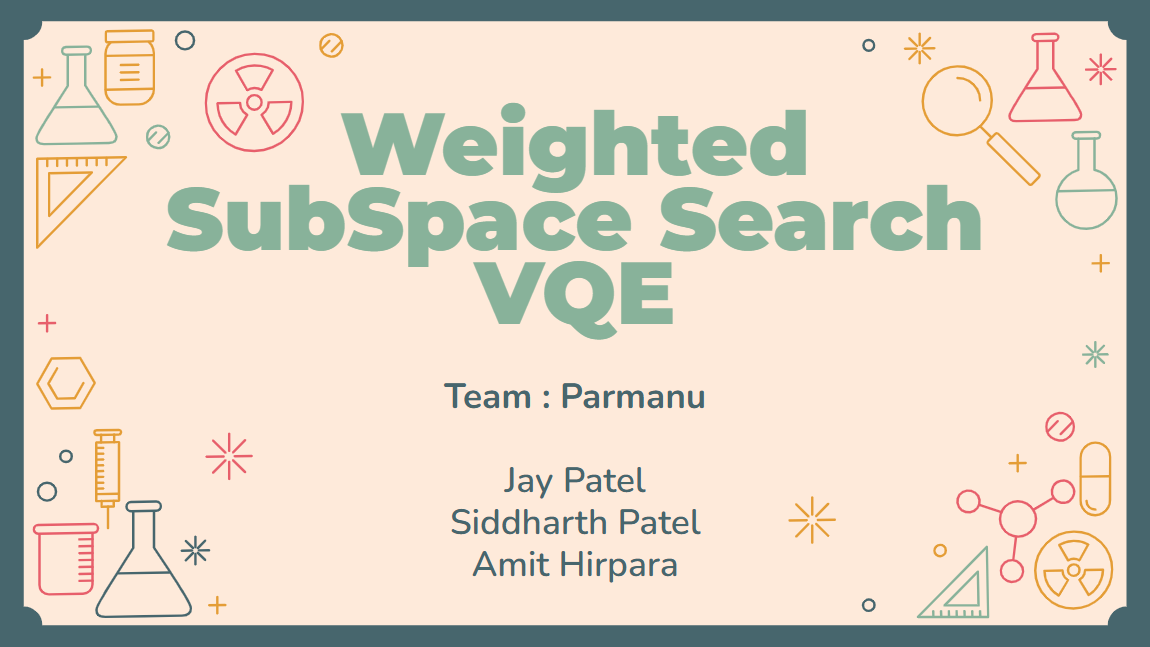
Demo information
Title Weighted SubSpace VQE to find Kth excited state energies
Abstract Generally, The variational quantum eigensolver (VQE) is used for finding the ground state energy for a given hamiltonian. To find the kth excited state energy of the hamiltonian we would need to run the VQE optimization process for at least k+1 time. Not to mention each time we need to calculate the hamiltonian again taking into account the state of the previous iteration. Even after that, the accuracy decreases as the value of k increases.
This is where the idea of Subspace Search VQE (SSVQE) comes in. The algorithm is used to find kth excited-state energy of a hamiltonian in just two subsequent optimization processes. The original research about the development of SSVQE is given in this paper. But, can we do better? Yes, the research shows that using the weights as hyperparameters we can find the kth excited-state energy in just a single optimization process. This is a more generalised version of SSVQE namely, Weighted SSVQE and it will be the centre of our focus in this project. There are two variants of this algorithm:
1) Weighted SSVQE to find kth excited state energy.
2) Weighted SSVQE to find all energies up to the kth excited state.
Relevant links Jupyter Notebook of the actual demo Same Notebook on Google Colab Reference Paper Molecular Structures