Closed yukyon closed 2 years ago
Judging from the file name, I suppose you take this model face_detection_yunet_2022mar
from opencv zoo. The one in this repo is the latest version of YuNet, and the one in opencv zoo is old version but we will update soon.
I suggest you take a look at https://github.com/ShiqiYu/libfacedetection.train/blob/master/onnx/yunet_yunet_final_dynamic_simplify.onnx. It is the latest YuNet and has dynamic shape.
As you said, using the yunet_yunet_final_dynamic_simplify.onnx model, I made a tflite model of the desired size.
However, an error occurs in the process of calculating the iou output during TFLite inference.
The reason is because of the strided_slice operation of TFLite. Below is a sample code written based on the yunet model for better understanding.
import tensorflow as tf
t = tf.constant([[[1, 1, 1], [2, 2, 2]],
[[3, 3, 3], [4, 4, 4]],
[[5, 5, 5], [6, 6, 6]]])
tf.strided_slice(
t,
begin=[0,0,-1],
end=[1,2,-1],
strides=[1,1,1],
begin_mask=2,
end_mask=2,
ellipsis_mask=0,
new_axis_mask=0,
shrink_axis_mask=0,
var=None,
name=None
)
result:
<tf.Tensor: shape=(1, 2, 0), dtype=int32, numpy=array([], shape=(1, 2, 0), dtype=int32)>
As a result of netron, it seems that shape=(1,2,1) should come out. As you can see, it is calculated as shape=(1, 2, 0) and no output is assigned.
Could you please review this issue? Could you make it like the output of the previous model?
Below is the last part of the converted tflite model graph.
ps. This could be a problem with converting slice to tflite's stride_slice. However, there is no way to fix it. I really want to try yunet.
I think you should file an issue on the repository of the convertion tool you used. As what I can see here, there is another possible solution: remove the slice part from the ONNX model.
So the slice nodes in the ONNX model come from the following lines: https://github.com/ShiqiYu/libfacedetection.train/blob/e5c4a24de8446bd1069b923a6f318d6602677ebd/model/yudet.py#L49-L56
Line 53 to line 55 correspond to the slice nodes in the ONNX model. You can comment out these lines, change the output and do some changes in export2onnx.py
to get your own ONNX model without slice nodes:
https://github.com/ShiqiYu/libfacedetection.train/blob/e5c4a24de8446bd1069b923a6f318d6602677ebd/export2onnx.py#L48
This problem seems to be a bug in the converter I used. As you said, I fixed the problem by modifying the output of yunet.py. ` class YuDetectNet(nn.Module): ...
def forward(self, x):
self.img_size = x.shape[-2:]
feats = self.backbone(x)
outs = self.head(feats)
head_data=[(x.permute(0, 2, 3, 1).contiguous()) for x in outs]
head_data = torch.cat([o.view(o.size(0), -1) for o in head_data], dim=1)
head_data = head_data.view(head_data.size(0), -1, self.out_factor)
loc_data = head_data[:, :, 0 : 4 + self.num_landmarks * 2]
conf_data = head_data[:, :, -self.num_classes - 1 : -1]
iou_data = head_data[:,:, head_data.shape[2]-1:head_data.shape[2]]
m = torch.nn.Softmax(dim=2)
conf_data = m(conf_data)
output = (loc_data, conf_data, iou_data)
return output
...
` The converter did not properly handle slices of indices containing negative numbers like head_data[:,:, -1:], so I modified it to slice with positive indices temporarily, bypassing the problem.
As a result, the converted tflite model worked well. Thank you very much for your great help.
Hello, I'm kijoong lee, a LG Electronics SW Developer.
We are developing a TFLite-based hardware-accelerated AI inference framework on webOS.
Recently, we judged YuNet to be the most suitable for face detection models through benchmarks. And, by converting the face_detection_yunet_2022mar.onnx model included in opencv dnn into a tflite model, a face detector with good performance was obtained. For reference, we used the xnnpack accelerated method.
However, we need a model larger than 160x120 that can be accelerated by GPU(or NPU), so we tried to convert the model included in https://github.com/ShiqiYu/libfacedetection.train/tree/master/onnx and use it, but it didn't work. .
The reasons we analyzed are as follows.
(face_detection_yunet_2022mar_float32.tflite)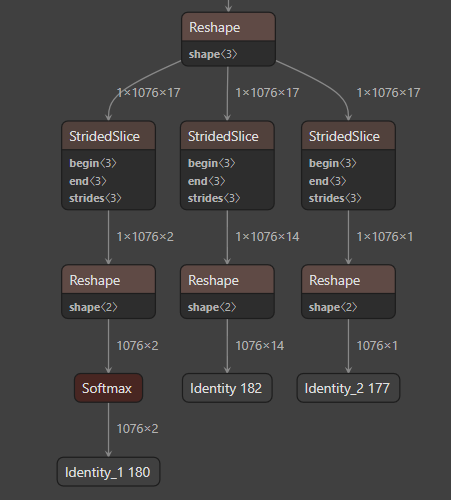
(yunet_yunet_final_320_320_simplify_float32.tflite)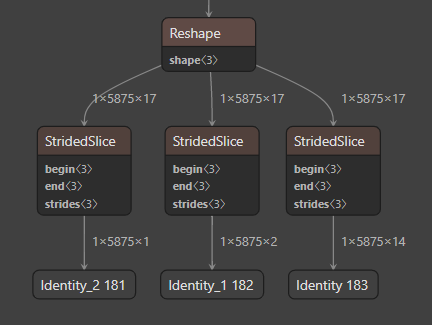
As you can see in the two figures above, the output shapes of the two models are different. A well-behaved model includes a reshaping part into a two-dimensional tensor and a Softmax operation.
How can we make a model with an input size larger than face_detection_yunet_2022mar.onnx? Or could you please fix this problem?