Astrobee Research CISC 489
Steven Kuntz, Colby Banbury, Evan DeAngelis
#
Abstract

Subjects, Methods, and Analysis
- NASA has the Astrobee Robot Software listed on Github
- The software uses ROS in order to operate the simulation and handle the image collection
- Using genetic algorithms we can provide ros commands to move the simulated astrobee to the desired coordinate location
- The Astrobee uses a coordinate positioning system that allows it to know it's position on the ISS at all times. We plan to use this position data to label images taken from the astrobees camera suite. This will be our training dataset for an genetic algorithm that, hopefully, will be able to detect the position of the astrobee from image data alone.
Research and Purpose
https://ntrs.nasa.gov/archive/nasa/casi.ntrs.nasa.gov/20160007769.pdf
- Astrobees used for three scenarios: research, camera, and search
- Allows for autonomous research, monitoring, and location of tools on the ISS
https://www.cc.gatech.edu/faculty/ashwin/papers/er-94-01.pdf
- Genetic algorithms can be used to teach a robots its paths to take for its destination
- good for unsupervised learning
https://www.analyticsvidhya.com/blog/2017/07/introduction-to-genetic-algorithm/
- Genetic algorithms: How they work and how to properly apply them
- Very useful for coming up with a road map for a future genetic algorithm for our application
Results
Initial Gazebo simulation

RVIZ Simulation

Depth Camera and Area Mapper

Image From Astrobee Camera

Astrobee Movement from ROS Commands

Conclusions
Over the course of this project, we had refine our end goal a lot. Initially we expected to be working with object detection of the astrobee and navigation of the robot. We underestimated the difficulty of deciphering software written by an outside party to be able to use as we pleased. Between set up and getting the data we needed, time was not in our favor to tackle it all. By the end of this project we accomplished:
- Setting up and running the simulation software
- Accessing the saved images from the simulator
- Finding the location data that corresponds with the image received
Direction for further Research
Genetic algorithms steps and how we would implement them
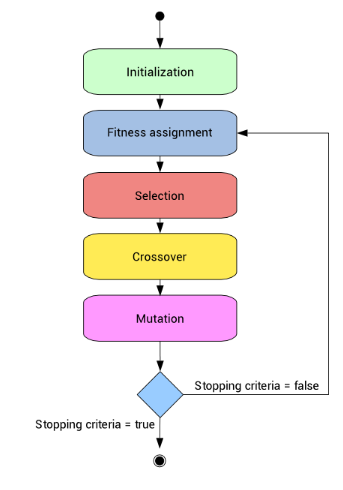
- Initialization
- Dataset:
- Create an image dataset and label each image with the corrispoinding location data from simulation
- Initial Population of detection algorithms:
- Create a population of different, rudimentary location detection algorithms based on existing image recognition software
- This will likely be the hardest stage as this part of Genetic algorithms does not easily work with image detection
- Fitness Function
- Define what is more fit:
- the closer the guess is to the actual coordinates the more fit the algorithm
- Selection
- Weighted Selection Criteria:
- Use the fitness from coordinate data to increase the chance of reproduction
- Source of randomness:
- Roulette Wheel Selection
- Fitness increases the chance but reproduction is still ultimately rando
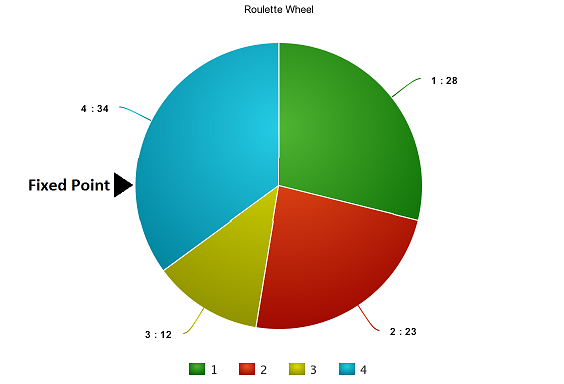
- Crossover
- Mix the traits of the parents
- Could increase ammount of crossover based on relative fitness
- Mutation
- Add in random weighting
- change the weighting of a certain aspect the image detection in some of the offspring