Closed eliahuhorwitz closed 3 years ago
@eliahuhorwitz looks like you are doing single node, single worker training so this isn't likely a concern, but you should be aware if you do distributed train, you should always confirm your validation results on a single node with the validation script afterwards, the distributed validation results will be a bit different due to padding of the batch, etc.
You can always double check the sanity of the bits and tpu results by using the same checkpoints and validating on a GPU with the the master branch, that is well tested.
For the official CIFAR training the vit authors used 98% of the train split https://github.com/google-research/vision_transformer/blob/main/vit_jax/configs/common.py#L93 ... unfortunately you can split 98 and then split again for multi-node train due to limitations in splitting up the samples for even distribution across distributed nodes right now.
The other factor is that the default weights are the ImageNet-21k 300epoch variants from the 'How to train your ViT' paper, not the original, 94.1 is the CIFAR-100 result for that paper for L/16 and 93.2 for B/16, L32 wasn't used, but the R50+L/32 hybrid had 93.9. Augmentation was off for the transfer runs in that paper.
One of the main observations in that paper was that when pre-training with higher augmentation + regularization w/ vision transformers, the results roughly match using an order of magnitude more data ... so in1k -> 21k and 21k -> jft300m as compared to the original paper.. thus your resutls aren't that crazy.
Hey @rwightman, thanks for the quick response!
you should always confirm your validation results on a single node with the validation script afterwards
Generally speaking, I do use distributed training. What would I need to do in order to change the validation to work on a single node? Also, would this still be needed for larger datasets (i.e ImageNet1k?)
You can always double check the sanity of the bits and tpu results by using the same checkpoints and validating on a GPU with the the master branch, that is well tested.
I'll try this! Is it also possible to move the validation to the CPU and run it on the master code? That way I can incorporate it into my training rather than manually switching machines?
unfortunately you can split 98 and then split
I'm assuming you meant "unfortunately you can't split 98 and then split"? In any case, this 2% split shouldn't make that much of a difference, right?
The other factor is that the default weights are the ImageNet-21k 300epoch variants from the 'How to train your ViT' paper, not the original, 94.1 is the CIFAR-100 result for that paper for L/16 and 93.2 for B/16, L32 wasn't used, but the R50+L/32 hybrid had 93.9. Augmentation was off for the transfer runs in that paper.
I was sure I was using the original ImageNet 30 epochs used in ViT, not the one from 'How to train your ViT'. Furthermotr, when I looked at 'How to train your ViT, I assumed these the VTAB results are on VTAB 1k, not the entire VTAB dataset 😓 (and hence I should compared them to the ones from the original ViT paper for example as seen below)
Also, is it possible to get the hyperparams used to train this (and other "best models" as you refer to them in the paper)? And do you happen to recall roughly how long would it take to train ViT-B/32 or L/32 on ImageNet1k and ImageNet21k?
The other factor is that the default weights are the ImageNet-21k 300epoch variants from the 'How to train your ViT' paper, not the original, 94.1 is the CIFAR-100 result for that paper for L/16 and 93.2 for B/16, L32 wasn't used, but the R50+L/32 hybrid had 93.9. Augmentation was off for the transfer runs in that paper.
Wouldnt 94.44 still be too high for using just the above script? This puts it almost on par with ViT-H/14 and almost at 2nd place for CIFAR100 SOTA.
So just to sum up, apart from maybe some small difference based on distributed validation, you don't think these high result are due to bug or some faulty handling of TPU training on my end?
Thanks again for the detailed and blazing fast response!
@eliahuhorwitz it is on the high side for L32, and yeah the L32 weights should still be the older 21k ones since there were no L32 weights for the new paper.
However, some of the L16 results for the new paper are well past 95 test accuracy in the index.csv in so it's not completely insane for good runs to be possible, you can explore all the detail of the pretrain hparams + transfer looking at the index csv used in the notebook linked below. The R50+L32 CIFAR100 highest test accuracy is listed ast 94.6 ...
I'm also not 100% clear why the VTAB table is different, if those are from a different train/val split than the index.csv transfer rseults. Lots of variables.
Yeah, using the :98% split, and then splitting that across N distributed nodes doesn't work right now.
Doing val on one node can be challenging, esp on TPU where all nodes need to do the same thing, I've tried setting up barriers and can be a bit flakey with hangs or timeouts on the idle nodes. Could probably run the same eval on all of them and throw the results out for all but rank 0. In either case, it's easier to just verify if there are any problems but running validation in isolation on a single CPU or GPU device to confirm no problems with your code or my bits and tpu code for this case. I've certainly used it a lot for imagenet and larger.
@eliahuhorwitz Looking at this while fixing #961, there is no problem at all here, the validation is correct. None of the problematic situations I was concerned about can arise with num_devices=1
and workers=1
, and even if they did, the impact would be smaller than the diffefrence between what you expected and what you got.
I ran your train command with default train script, tfds dataset, reproduced your accuracy numbers per epoch exactly. Nice to see the reproducability.
I exported just the state dict (clean_checkpoint.py) so it's loadable in the master branch, and ran on GPU with torch cifar and tfds and both were within .02 of the original train val. 94.17 vs 94.19 which is well within expected varations across hardware types.
So the reason, most likely that you are fine tuning from the in1k fine tuned 384x384 weights and not the 224x224 in21k weights as in the paper. You can do the later, use vit_large_patch32_224_in21k
but you do have to hand code an img_size=384
into the create_model since only the vit and mlp models accept and img_size arg, it will then interpolate pos embed for you.
Another possibility is that the authors were using 98% of train split for train, :2% for eval (checkpoint selection), and then final test was evaluated once. It wasn't clear from the first paper if that was the case or not. But I know this group likes to avoid doing eval on the test and sometimes make their own val splits when there are none.
When I was working with the vit authors on the How to train your vit
paper, I pointed out that I'd found transfer results were better from the 21k-1k->other target dataset than from 21k->other dataset. It wasn't explored much as it wasn't the focus of that paper.
I'm closing this and will move on to fixing #961 with the checkpoints I have and will add some tweaks for the split handling in tfds and torch dataset support for this and a few other datasets.
@rwightman Terrific, thanks! Also, it may be worth addining some clarity in the README or documentation regarding the different ViT checkpoints and how they may differ drastically from the figures in the paper. I started by reproducing the numbers from the paper, and once I got them I started changing things comparing my result to that baseline. If you want, I am happy to write something and open a PR
@eliahuhorwitz It'd be helpful to have some info, yes but I'm not exactly sure where it'd go (to be obvious), and how to write it to provide clarity instead of more confusion. It feels like a non-trivial effort to fully cover the different checkpoints (there are those in timm as the defaults and also many that exist outside of timm that can be loaded via .npz files from the google repo). I've got a million other tasks to get through so not a high priority for me at the moment. Open to a PR if adds clarity, but don't know quite where to put it...
Hey, I've been finetuning ViT on different datasets (cifar100, oxford_pets, etc.). I am using Google TRC TPUs, specifically V3 VM using the bits_and_tpu branch. I have found the results of finetuning to be odd, specifically, on CIFAR100 I am seeing the eval top1 accuracy reaching 94.19 within 17 epochs (I even had 1 run get to 94.44), these numbers are closer to JFT300 results and not ImageNet21K results. From the original ViT paper below they get 93.04 on a similar setup to mine and from the google research github repo also attached below the get 93.29. Even more surprising to me is the fact I get the 94.x results when I turn off the image augmentations.
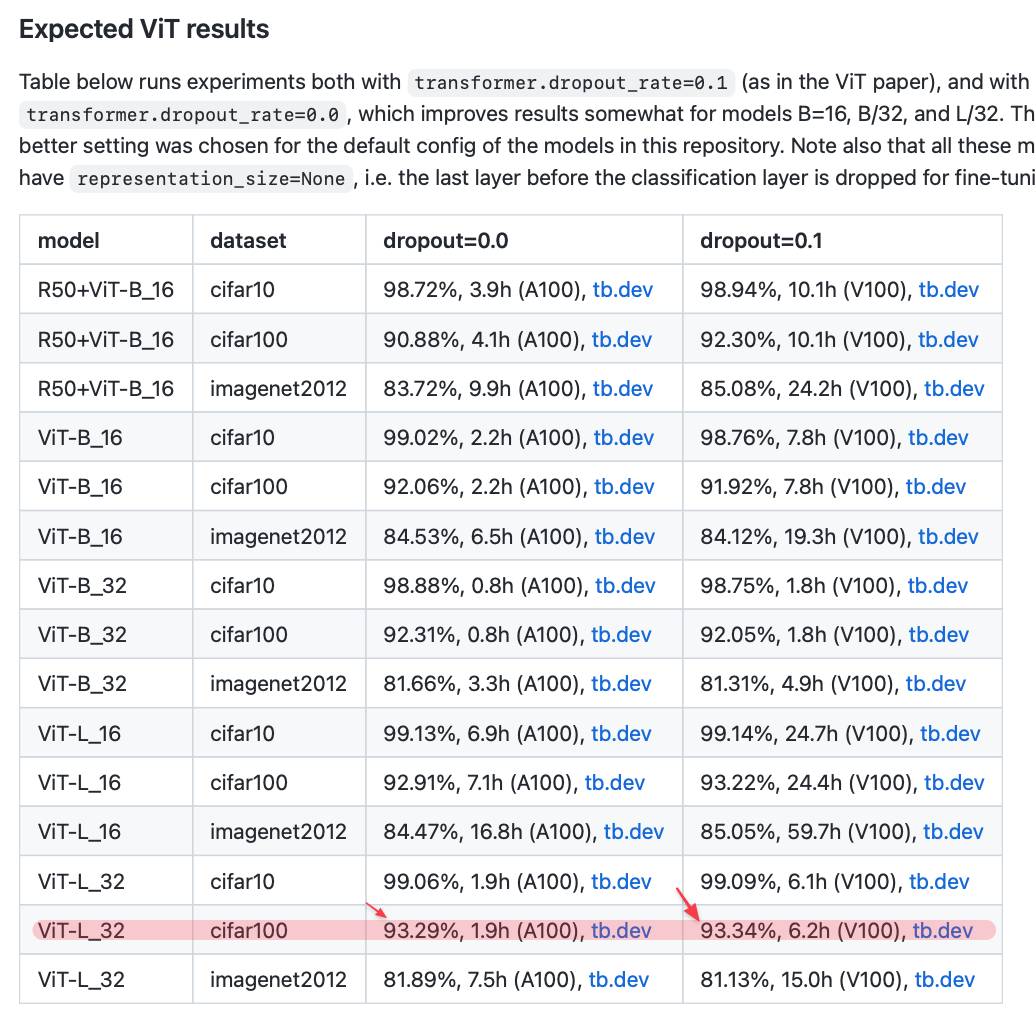
To try and ensure I didn't introduce a bug into the codebase, I cloned a new copy of the repo and performed tests aginst it. I start finetunning with:
python3 launch_xla.py --num-devices 1 finetune.py ~/tensorflow_datasets --dataset tfds/cifar100:3.0.2 --opt sgd --epochs 1000 --workers 1 --val-split test --mixup 0 --cutmix 0 --opt-eps=1e-08 --train-interpolation=bicubic --warmup-lr=1e-06 --lr 0.004 -b 128 --num-classes 100 --model vit_large_patch32_384
and my finetune.py file is just a copy of the train script with a change in the way I create the mode, that is I comment out this
and instead put this
model = timm.create_model(args.model, pretrained=True, num_classes=args.num_classes)
The full script is below:
Here is the summary of the above output (I stopped it once I saw it is too high)
And here is a graph of a similar run with slightly different hyperparams which I let run for longer (it reached 94.44!!!)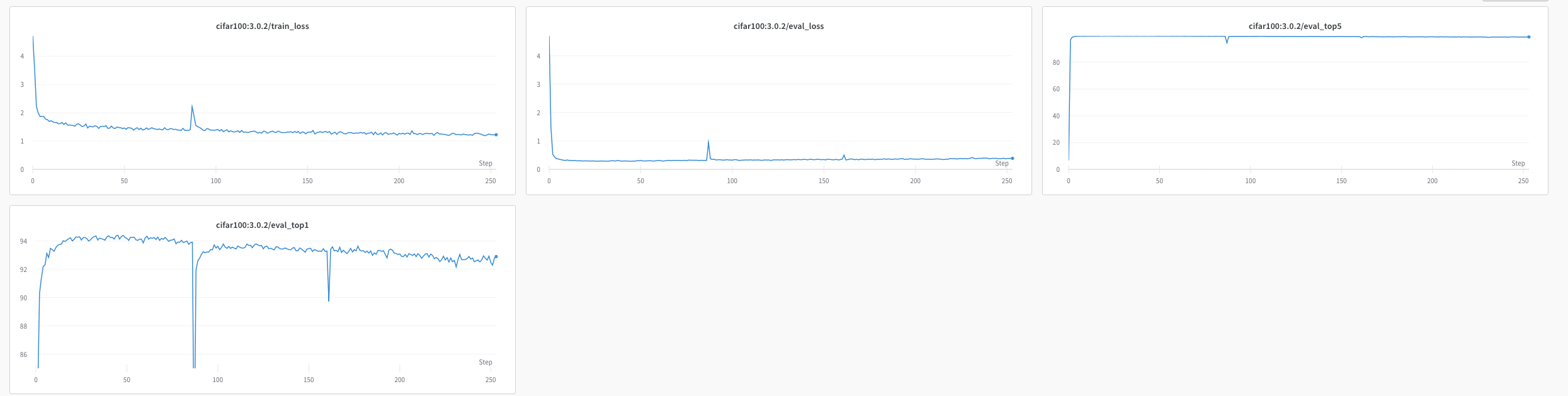
I've made sure to start a clean machine for this, with a fresh download of cifar100 from TFDS, and of course, a fresh clone of the codebase.
The above results also make me completely doubt the results I have been getting for my own models that use this codebase/pretrained models. I am working now on trying to reproduce this on a GPU, but I don't have access to the same amount of compute so this is going to be more challenging.
Am I somehow missing something or doing something wrong in the fine-tuning script? Could these be real results? Or do you think there is some bug in the XLA/TPU side of things?
Do you have any recommendations as to where should I start looking for a solution?
Thanks, Eliahu