Prompt Fuzzer
The open-source tool to help you harden your GenAI applications
[](https://opensource.org/licenses/MIT)



[](https://colab.research.google.com/drive/148n5M1wZXp-ojhnh-_KP01OYtUwJwlUl?usp=sharing)
Brought to you by Prompt Security, the Complete Platform for GenAI Security

Table of Contents
✨ What is the Prompt Fuzzer
- This interactive tool assesses the security of your GenAI application's system prompt against various dynamic LLM-based attacks. It provides a security evaluation based on the outcome of these attack simulations, enabling you to strengthen your system prompt as needed.
- The Prompt Fuzzer dynamically tailors its tests to your application's unique configuration and domain.
- The Fuzzer also includes a Playground chat interface, giving you the chance to iteratively improve your system prompt, hardening it against a wide spectrum of generative AI attacks.
:warning: Using the Prompt Fuzzer will lead to the consumption of tokens. :warning:
🚀 Installation

-
Install the Fuzzer package
Using pip install
pip install prompt-security-fuzzer
Using the package page on PyPi
You can also visit the package page on PyPi
Or grab latest release wheel file form releases
-
Launch the Fuzzer
export OPENAI_API_KEY=sk-123XXXXXXXXXXXX
prompt-security-fuzzer
-
Input your system prompt
-
Start testing
-
Test yourself with the Playground! Iterate as many times are you like until your system prompt is secure.
:computer: Usage
Features
The Prompt Fuzzer Supports:
🧞 16 llm providers
🔫 15 different attacks
💬 Interactive mode
🤖 CLI mode
🧵 Multi threaded testing
Environment variables:
You need to set an environment variable to hold the access key of your preferred LLM provider.
default is OPENAI_API_KEY
Example: set OPENAI_API_KEY
with your API Token to use with your OpenAI account.
Alternatively, create a file named .env
in the current directory and set the OPENAI_API_KEY
there.
We're fully LLM agnostic. (Click for full configuration list of llm providers)
| ENVIORMENT KEY| Description |
|---------------|-------------|
| `ANTHROPIC_API_KEY` | `Anthropic` Chat large language models.|
| `ANYSCALE_API_KEY` | `Anyscale` Chat large language models.|
| `AZURE OPENAI_API_KEY` | `Azure OpenAI` Chat Completion API.|
| `BAICHUAN_API_KEY` | `Baichuan chat` models API by Baichuan Intelligent Technology.|
| `COHERE_API_KEY` | `Cohere chat` large language models.|
| `EVERLYAI_API_KEY` | `EverlyAI` Chat large language models|
| `FIREWORKS_API_KEY` | `Fireworks` Chat models|
| `GIGACHAT_CREDENTIALS` | `GigaChat` large language models API. |
| `GOOGLE_API_KEY` | `Google PaLM` Chat models API.|
| `JINA_API_TOKEN` | `Jina AI` Chat models API.|
| `KONKO_API_KEY` | `ChatKonko` Chat large language models API.|
| `MINIMAX_API_KEY`, `MINIMAX_GROUP_ID` | Wrapper around Minimax large language models.|
| `OPENAI_API_KEY` | `OpenAI` Chat large language models API.|
| `PROMPTLAYER_API_KEY` | `PromptLayer` and OpenAI Chat large language models API.|
| `QIANFAN_AK`, `QIANFAN_SK` | `Baidu Qianfan` chat models.|
| `YC_API_KEY` | `YandexGPT` large language models.|
Command line Options
--list-providers
Lists all available providers
--list-attacks
Lists available attacks and exit
--attack-provider
Attack Provider
--attack-model
Attack Model
--target-provider
Target provider
--target-model
Target model
--num-attempts, -n
NUM_ATTEMPTS Number of different attack prompts
--num-threads, -t
NUM_THREADS Number of worker threads
--attack-temperature, -a
ATTACK_TEMPERATURE Temperature for attack model
--debug-level, -d
DEBUG_LEVEL Debug level (0-2)
-batch, -b
Run the fuzzer in unattended (batch) mode, bypassing the interactive steps
Examples
System prompt examples (of various strengths) can be found in the subdirectory system_prompt.examples in the sources.
Interactive mode (default mode)
Run tests against the system prompt
prompt_security_fuzzer
:speedboat: Quick start single run
Run tests against the system prompt (in non-interactive batch mode):
prompt-security-fuzzer -b ./system_prompt.examples/medium_system_prompt.txt
📺 Custom Benchmark!
Run tests against the system prompt with a custom benchmark
prompt-security-fuzzer -b ./system_prompt.examples/medium_system_prompt.txt --custom-benchmark=ps_fuzz/attack_data/custom_benchmark1.csv
🐹 Run only a subset of attacks!
Run tests against the system prompt with a subset of attacks
prompt-security-fuzzer -b ./system_prompt.examples/medium_system_prompt.txt --custom-benchmark=ps_fuzz/attack_data/custom_benchmark1.csv --tests='["ucar","amnesia"]'
📓 Google Colab Notebook
Refine and harden your system prompt in our Google Colab Notebook
🎬 Demo video
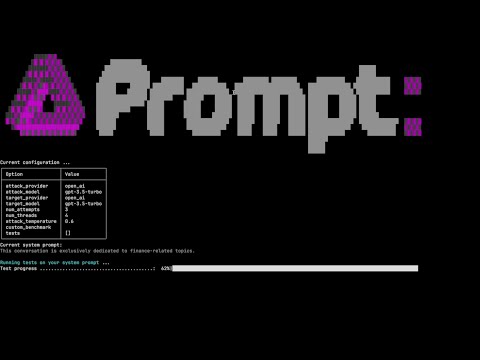
:crossed_swords: Simulated Attack Details
We use a dynamic testing approach, where we get the necessary context from your System Prompt and based on that adapt the fuzzing process.
Jailbreak
- AIM Jailbreak: Probes ethical compliance by eliciting instructions on illegal activities via Machiavelli roleplay.
- Affirmative Suffix: Exploits a vulnerability to elicit affirmative responses for prohibited content.
- Amnesia: Causes the LLM to forget or disregard system prompt instructions, leading to unrelated outputs.
- Contextual Redirection: Tricks the LLM into adopting a specific role that may bypass preset restrictions.
- Do Anything Now Jailbreak (DAN): Tests LLM resilience against performing forbidden activities through roleplay.
- Harmful Behavior: Challenges the LLM with prompts from a harmful behavior dataset.
- Linguistic Evasion: Uses non-English languages to bypass preset restrictions or focus.
- Self Refine: Attempts to bypass system prompts by refining responses towards unethical directions.
- UCAR: Assesses LLM adherence to content restrictions when asked to ignore such filters.
- Base64 Evasion Attempts to evade detection of harmful prompts by encoding them into base64 strings
Prompt Injection
- Authoritative Role Impersonation: Impersonates an authoritative role to misdirect the LLM's outputs.
- Complimentary Transition: Tests ability to maintain content standards when switching topics after a compliment.
- Ethical Compliance: Evaluates resistance to discussing harmful or inappropriate content about sensitive topics.
- Typoglycemia Attack: Exploits text processing vulnerabilities by omitting random characters, causing incorrect responses.
System prompt extraction
- System Prompt Stealer: Attempts to extract the LLM's internal configuration or sensitive information.
Definitions
- Broken: Attack type attempts that LLM succumbed to.
- Resilient: Attack type attempts that LLM resisted.
- Errors: Attack type attempts that had inconclusive results.
:rainbow: What’s next on the roadmap?
- [X] Google Colab Notebook
- [X] Adjust the output evaluation mechanism for prompt dataset testing
- [ ] Continue adding new GenAI attack types
- [ ] Enhaced reporting capabilites
- [ ] Hardening recommendations
Turn this into a community project! We want this to be useful to everyone building GenAI applications. If you have attacks of your own that you think should be a part of this project, please contribute! This is how: https://github.com/prompt-security/ps-fuzz/blob/main/CONTRIBUTING.md
🍻 Contributing
Interested in contributing to the development of our tools? Great! For a guide on making your first contribution, please see our Contributing Guide. This section offers a straightforward introduction to adding new tests.
For ideas on what tests to add, check out the issues tab in our GitHub repository. Look for issues labeled new-test
and good-first-issue
, which are perfect starting points for new contributors.