## :computer: Online RNA-MSM Sever
We also built a freely accessible [web server](https://aigene.cloudbastion.cn/#/rna-msm) for using the RNA-MSM models, You may effortlessly submit tasks onto the server and subsequently receive the outcomes via email, without the need to configure the environment or consume any computational resources.
As a preview, take a swift glance at the website:
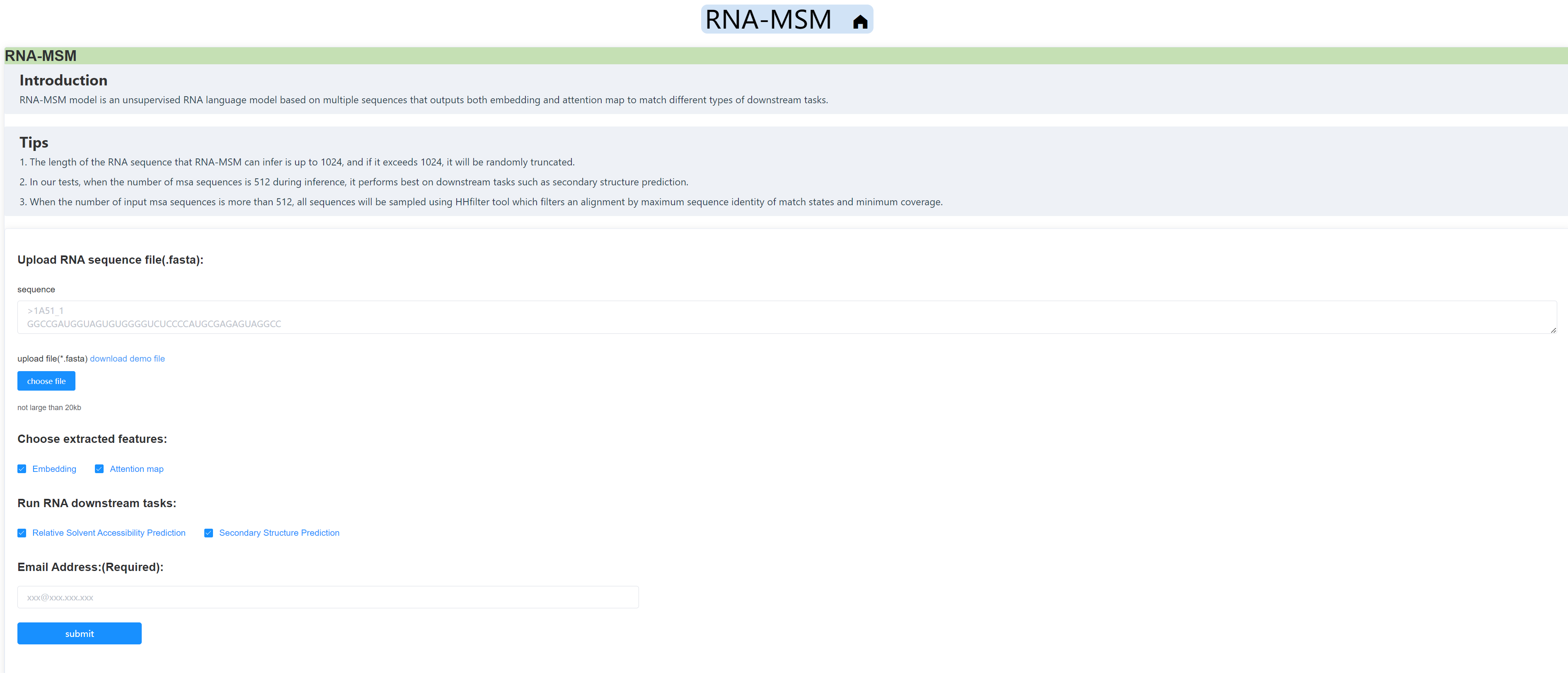
## :pushpin: Citation
If you find our work useful in your research or if you use parts of this code please consider citing our paper:
```
@article{10.1093/nar/gkad1031,
author = {Zhang, Yikun and Lang, Mei and Jiang, Jiuhong and Gao, Zhiqiang and Xu, Fan and Litfin, Thomas and Chen, Ke and Singh, Jaswinder and Huang, Xiansong and Song, Guoli and Tian, Yonghong and Zhan, Jian and Chen, Jie and Zhou, Yaoqi},
title = "{Multiple sequence alignment-based RNA language model and its application to structural inference}",
journal = {Nucleic Acids Research},
volume = {52},
number = {1},
pages = {e3-e3},
year = {2023},
month = {11},
abstract = "{Compared with proteins, DNA and RNA are more difficult languages to interpret because four-letter coded DNA/RNA sequences have less information content than 20-letter coded protein sequences. While BERT (Bidirectional Encoder Representations from Transformers)-like language models have been developed for RNA, they are ineffective at capturing the evolutionary information from homologous sequences becauseĀ unlike proteins, RNA sequences are less conserved. Here, we have developed an unsupervised multiple sequence alignment-based RNA language model (RNA-MSM) by utilizing homologous sequences from an automatic pipeline, RNAcmap, as it can provide significantly more homologous sequences than manually annotated Rfam. We demonstrate that the resulting unsupervised, two-dimensional attention maps and one-dimensional embeddings from RNA-MSM contain structural information. In fact, they can be directly mapped with high accuracy to 2D base pairing probabilities and 1D solvent accessibilities, respectively. Further fine-tuning led to significantly improved performance on these two downstream tasks compared with existing state-of-the-art techniques including SPOT-RNA2 and RNAsnap2. By comparison, RNA-FM, a BERT-based RNA language model, performs worse than one-hot encoding with its embedding in base pair and solvent-accessible surface area prediction. We anticipate that the pre-trained RNA-MSM model can be fine-tuned on many other tasks related to RNA structure and function.}",
issn = {0305-1048},
doi = {10.1093/nar/gkad1031},
url = {https://doi.org/10.1093/nar/gkad1031},
eprint = {https://academic.oup.com/nar/article-pdf/52/1/e3/55443207/gkad1031.pdf},
}
```
## :phone: Contact
Yikun Zhang - yikun.zhang@stu.pku.edu.cn